Don't be April Fooled by AI
It's temping to think ChatGPT and its AI friends can answer all of your questions well. But their true power is getting you to ask the question. Read: "Everything Starts Out Looking Like a Toy" #192
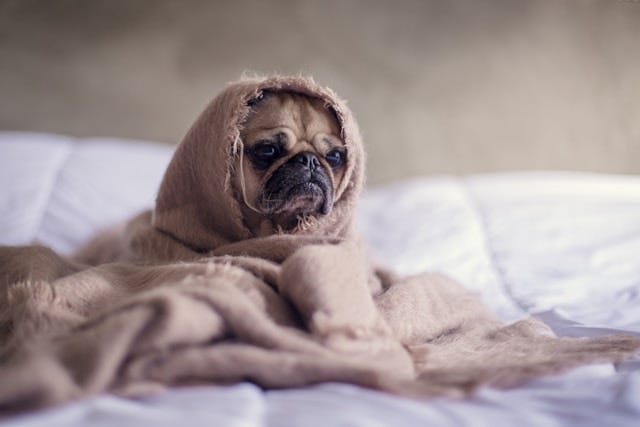
Hi, I’m Greg 👋! I write weekly product essays, including system “handshakes”, the expectations for workflow, and the jobs to be done for data. What is Data Operations? was the first post in the series.
This week’s toy: integrating a rotary phone to make calls on today’s networks. What’s great about this how-to is that it has more software than hardware. The outcome: an old-school rotary phone that can make calls to any other phone. I often think about building one of these DIY cell phone kits but am not confident all the parts would end up together correctly. Edition 192 of this newsletter is here - it’s April 1, 2024.
Brought to you by Apollo.io, an all-in-one solution providing RevOps teams with access to data, enrichment, outreach, call intelligence, scoring, calendaring, and email automation. They integrate seamlessly with your CRM and existing workflows. The quality of Apollo’s data is unmatched, they are ranked #1 for contact and company data accuracy on G2 with over 6000 reviews and a 4.8 star rating. Learn more …
If you have a comment or are interested in sponsoring, hit reply.
The Big Idea
A short long-form essay about data things
⚙️ Don’t be April Fooled by AI
I’d be remiss on April 1st for not thinking about the head-fakes we all create for each other (mostly for fun). Today, some of these distractions will be created by ChatGPT or other AI tools, and many will be quite believable. You’re good at being cautious and can probably detect the obvious fakes. But what about the ways that you could be using AI to help with your ops tasks?
It’s easy to start with the extreme assumption that AI will either be exactly no help to the operations professional or that it will automate away many of those tasks. The likely outcome is going to be somewhere in between, which is exactly why it’s important to think about the areas where AI can be a game changer for data operations.
Let the computers count
AI (or even machine learning tasks) are great at counting and finding trends in data. One place to use AI for operations is to analyze a very large dataset with a data quality question in mind.
These questions look like:
How many accounts follow the pattern of logging in one day and then being unresponsive?
What’s the average sales cycle for accounts that started in January?
It would be nice if AI could do this work seamlessly by simply answering this question. It turns out the context for the AI/ML/Analyst creates the important work that we do in operations, even if the computers do more of the counting over time.
Context and guardrails are everything.
If you ask an LLM what kind of sandwich is possible with some ingredients, it’s no big deal if you get it wrong.
If you ask a model to check a financial statement and validate that the numbers foot, there is a much bigger consequence for getting it wrong, and specialized knowledge is needed to provide a correct answer that will stand up to scrutiny.
Don’t get fooled by AI into thinking that it will do the work for you. Do use queries, LLMs, and other tools to make it easy to find small mistakes that humans will easily miss.
Your greatest friend might be a query or a spreadsheet that shows no rows unless something needs to be fixed. AI models and LLMs are not good at seeing what is out of place until you tell them to match on that thing.
Build your Socratic Strength
Another way you can get April fooled by AI is by asking it a single question and expecting it to get the answer right in a one-shot response. There are many reasons why this doesn’t work well, but the main one is that context thing.
A model doesn’t know your Rules of Engagement.
A model doesn’t know how to handle a billing edge case.
A model doesn’t know how to design an email sequence that is right for your customers.
But AI does know how to answer questions. One of the best ways to avoid getting April Fooled by AI is to ask it to imagine a situation and start playing out what you would do when that happens.
For example:
“Brainstorm some approaches to asking people to start their paid subscriptions early.”
“Help me compose language to approach people when they have a past due invoice”
“Suggest 5 ways to measure the health of my pipeline and the velocity of revenue”
Your mileage may vary, and going through the process of asking and answering these questions will make you test your assumptions. The model won’t get the answers right. But you will have a better understanding of how to answer the question.
Getting Ready for an AI Practice
Sooner or later, using features powered by AI will be commonplace. The big breakthrough will not be that AI solves all problems, but rather that we will let AI do a lot of the things we formerly assigned to other machine driven procedures.
Whether the hook is “clean your data better”, “make predictions for which accounts will sell more quickly”, or “brainstorm some innovative ideas”, you’ll still need human smarts to evaluate these ideas and to determine whether they make sense for your business.
Many people have described the current level of AI progress to be “an eager intern” and that’s a good frame for thinking about the level of tasks you’ll be able to delegate (and validate) at this point in time.
You’ll need to figure this out. AI’s not going to remove the need for Operations Pros. But Operations pros who use AI and machine learning features will succeed over those who don’t use AI.
What’s the takeaway? It’s easy to be April fooled by the promise of AI. When it returns a blob of information that pattern matches on things we know to be true, the temptation is to assume that calculation is right. But until we have AGI, computers are dumb. They do what we tell them to do. That means that the context and procedure we use for AI is key for improving productivity and getting the answers (and questions) we want.
Links for Reading and Sharing
These are links that caught my 👀
1/ Hold data cautiously - This essay by Sean Coates might seem extremely privacy-focused. He writes that his company deliberately designed their systems to be unable to decode customer data. And it’s a fascinating look into product decisions that drive other product decisions. When your North Star is to maintain privacy at all costs, that gives a key tie-breaker to many decisions and makes product design much simpler.
2/ Applied Cryptography - One of the hardest things to read is a doctor’s signature. So why are we advocating to teach Cursive Writing again in schools? Writing activates a different part of your brain than typing, and applying cursive writing as a craft applies in lots of different ways.
3/ Get off the Ops Struggle Bus - Jeff Cullimore writes about breaking the struggle cycle for ops. He advocates that ops teams behave more like internal product teams to build a roadmap based on the biggest impact they can have for their company. “Internally-facing Product Manager” sounds more strategic than Ops Firefighter, so that’s a good place to aim.
What to do next
Hit reply if you’ve got links to share, data stories, or want to say hello.
Want to book a discovery call to talk about how we can work together?
The next big thing always starts out being dismissed as a “toy.” - Chris Dixon
I've thought a lot about how much asking bots questions structures my own thinking. It's an incredible force function and training on some healthy comms tactics with humans (if you do it well)