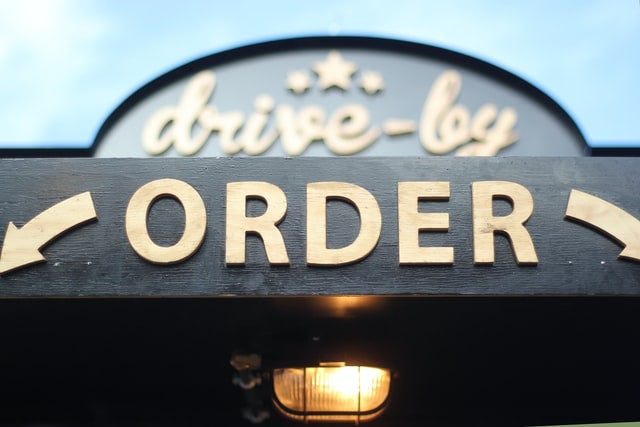
Welcome to the first 73 Find Data Ops 📊 members who have joined us since launch!
Join smart, curious folks by subscribing here:
Hi, I’m Greg 👋! I publish this newsletter on finding data products and interesting data observations with the goal of finding patterns and future product insights. (Also, it’s fun.) If you need a background on how we got here, check out What is Data Operations?
This week’s toy: a town-building game you play in your browser. Don’t take my word for it: try it out! I’m guessing you’ll have a hard time not clicking on things and seeing what happens. Edition No. 72 of this newsletter is here - it’s December 13, 2021.
The Big Idea
A short long form essay about data things
📊 Today’s Dashboards are broken.
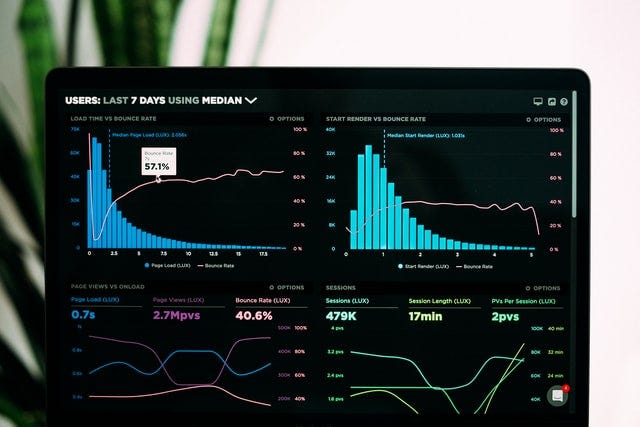
Dashboards are not doing well. The main reason? They tell the truth, and we don’t want to hear it. What I mean here is that dashboards deliver the result of our actions and they do so in the context of definitions that we *might* agree to later, but at the time they seem to make sense.
What’s the disconnect? Dashboards show us both our current performance and the historical results of that data. If you’ve read the book Thinking Fast and Slow, you might remember that Daniel Kahneman writes about “Type 1” and “Type 2” thinking – the comparison between faster, automatic thinking and slower, data-driven analysis – as a way to demonstrate that people overweight the current task.
We pay more attention to things right now because we’re not good at estimating (or remembering) how long things took in the past and we also assume that things will go forward without complication. What could possibly go wrong? Often times, a lot.
Dashboards Display the Truth
Dashboards make people nervous because they show the historical evidence. That might not be the same as everyone’s shared truth, but it is a record of the things that happened. When teams look at dashboards, usually disagreements are grounded in the analysis of that historical record. We don’t remember or note a whole lot about what was happening at the time that data series was captured.
A key reason we don’t write down what happened as it is happening is that it’s hard to get agreement on what to do to solve a complex problem. Think about this in the context of Sales and Marketing operational data. Most people agree on the definition of Annual Revenue: it’s the sum total of the closed won opportunities sold by the company. But when you start getting into the definition of a “qualified lead” or a “marketing qualified lead” or a “sales qualified lead”, things get contentious.
Maybe what we need for analytics is a better focus on the way we solve problems collaboratively.
Analytics as a Multi-player Game
Successful consumer applications work because they have few “jobs to be done” and users of that application want to get the job done. Whether it is searching for an address, finding a tasty meal, or buying something online, the outcome is relatively clear. You know you ordered a book when you get an email. You know that the food is tasty because you’re eating it.
Perhaps the future of analytics is “Yelp for the enterprise”, as coined by Benn Stancil. As Benn writes,
Yelp and Google work because they know exactly what they’re there to do. Yelp’s homepage, for instance, makes it very clear what it's for: Find things near you. There’s no “exploring data” or “discovering insights” on restaurants. Instead, Yelp presents a problem that needs data to be solved.
Stancil points out accurately that consumer apps make it easy to point the user at a funnel where the end of the funnel is an outcome of a decision.
You don’t have this in a business context because no one has agreed on the equivalent of “choosing to go for a meal.” Business dashboards make it easy to blend information into almost any format and even good dashboards are not terribly opinionated on what’s a good outcome.
When the base question has been posed, its easier to answer. How are we doing, specifically? Dashboards don’t often do this well because it’s hard to get the agreement on what to do to solve a complex problem.
A 3 Star Dashboard
In the analytics world today, we’re often focused on displaying information that’s happening rather than pointing on what needs to be done and what problem. This is sort of like a Yelp review with ⭐️⭐️⭐️ - “it was pretty good, but tbh I was extremely hungry at the time” - not exactly a ringing endorsement for excellent food.
To carry forward the metaphor, our Dashboards today show you lots of restaurants, don’t really tell you which ones are good to go to, and deliver aggressively bland data. This causes a disconnect. When you meet someone who’s a foodie and who finds the unexpected restaurant gems in a city or neighborhood, it puts everything else in perspective.
How can we provide that wow factor for business data?
A Dashboard you want to use …
A better dashboard wouldn’t just give you all of the information from the environment. It would do a great job of helping you to answer “what-if” questions that you want to solve that are relevant to improving your business. This means not only showing you how data relates and which ideas provide the most influence on a particular metric, but also making it easier to decompose that idea into plain English to tell a narrative story of what was happening at that point in time, not just a data point.
When you combine accurate notes or date sampling, they provide a “movie” of what was happening in the business instead of a single snapshot of data at one point in time.
The best dashboards capture the decisions we are making today and present them through time in a way that showcases the adjustments we make along the way. Forecasts start out as … well, forecasts. They are not actuals until they happen.
Our reporting should reflect that, by having:
definitions that are easily understood by everyone in the business
results and inputs shared by multiple people and departments, not limited to a single departmental system of record
easy to understand by anyone who works at the company
When we track these results over time and provide context, it will be easier to er time so that we combine these concepts of overweighting the value of the current task and understanding the historical evidence of what happened.
What’s the takeaway? Analytic systems must acknowledge they are evaluated by people, and therefore need to build in some signalling to counter the biases that people have. In particular it’s important to record the choices that we make so that when we review them later there’s valuable context to assess whether the decision was good (at the time) or good (even now).
A Thread from This Week
A Twitter thread to dive into a topic
If you’re stuck for ideas, look for successful outcomes in another industry - here are a few examples of cross-industry innovation.

Links for Reading and Sharing
These are links that caught my 👁
1/ Remote work is not always synchronous - If you want to get value from remote work, you need to specify what needs to be done, find the right people who are capable of getting that work done, and let them do it themselves. It takes a different set of tools and expectations to make remote work successful, not just selecting the digital analog of what you used to do in person.
2/ Using pricing choices to drive the business - pricing is one of the most important strategic levers for a business. This 2015 post from Eric Jorgenson still works as an excellent summary of the various choices involved. Saas execs should pay attention to the portion on setting a platform price and then later using that advantage to offer differential pricing based on the small things you want customers to do next.
3/ Remove drag to add speed - It’s easier to avoid mistakes than do something amazing. Consider this idea:
It is remarkable how much long-term advantage people like us have gotten by trying to be consistently not stupid, instead of trying to be very intelligent.
-Charlie Munger
Does this mean you should always take the conservative route to avoid crashing and burning? Not really, but it does suggest that some of your bets should be sure ones.
On the Reading/Watching List
Some things to watch or read, in no particular order
Reading: Better Data Visualizations: A Guide for Scholars, Researchers, and Wonks - a book by Jonathan Schwabish. Putting together visual presentations of data is often a challenge. How do you pick the right message, for the right audience, at the right time; and visualize it in pleasing way? One way is to look at great examples!
Watching: on the history of 16 bit music. That multi-track synthesized music you might remember or appreciate came from early computer and video games in the 1980s and 1990s. This documentary examines the beginning and structure of those sounds. Sega Genesis and Amiga game lovers, unite!
What to do next
Hit reply if you’ve got links to share, data stories, or want to say hello.
I’m grateful you read this far. Thank you. If you found this useful, consider sharing with a friend.
Want more essays? Read on Data Operations or other writings at gregmeyer.com.
The next big thing always starts out being dismissed as a “toy.” - Chris Dixon