Trust in Numbers: Building Reliable Reporting Through Cross-Functional Collaboration
Data and GTM teams need to work together to develop and deliver reliable, accurate, and relevant reporting. Read the third in a series: "Everything Starts Out Looking Like a Toy" #148
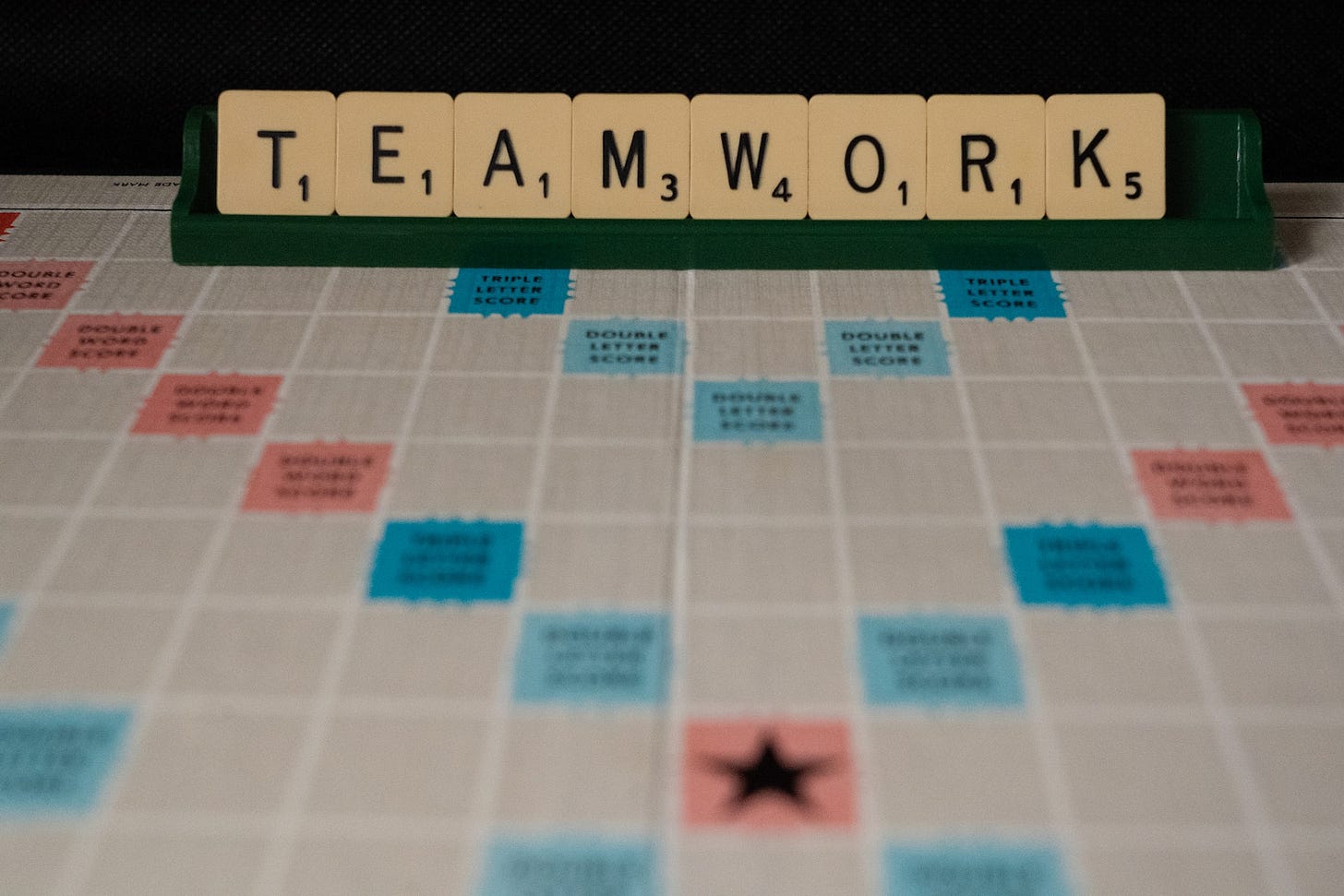
Hi, I’m Greg 👋! I write weekly product essays, including system “handshakes”, the expectations for workflow, and the jobs to be done for data. What is Data Operations? is a post that grew into Data & Ops, a team to help you with product, data, and operations.
This week’s toy: how to build a video booth to simulate the “bullet time” special effect from the Matrix. Wedding photography will never be the same. Edition 148 of this newsletter is here - it’s June 5, 2023.
Brought to you by Pocus, a Revenue Data Platform built for go-to-market teams to analyze, visualize, and action data about their prospects and customers without needing engineers. Pocus helps companies like Miro, Webflow, Loom, and Superhuman save 10+ hours/week digging through data to surface millions in new revenue opportunities.
If you're reading and haven't subscribed (yet), give it a try! And if you have any comments or are interested in sponsoring, hit reply.
The Big Idea
A short long-form essay about data things
⚙️ The Path to PLG Success: Data and GTM teams join forces to improve data and next actions
The second post in the Path to PLG Success series discussed the way that Data and GTM teams arrange their data to facilitate the structure, metrics, and update cadence to support the business. Remember, It's all about ensuring everyone's rowing toward the same goal with product-led growth to accelerate revenue.
Tl;dr: the three things we highlighted were building the database and the included data to support the needs of fast-changing data, defining metrics that make sense to the rest of the organization, and the ability to update that data quickly to support fresh data for the GTM system.
We’re all about finding ways to help data and GTM teams work better together and drive growth for their companies. For the next post, we’re discussing the way these teams create reliable reporting to communicate the metrics and data to the rest of the organization.
Trust in Numbers: Building Reliable Reporting Through Cross-Functional Collaboration
Businesses – especially Saas businesses – depend on reliable reporting to navigate the waters of their business. To define “reliability”, think about the component parts of that data.
For the rest of the business to believe that you’ve done a good job, the Data Team and the GTM team have to provide a dependable, consistent, and trustworthy source of data when they create reports.
There are many different kinds of reporting that you might build: for the purpose of this post, let’s focus on operational reporting that supports GTM metrics and goals. These reports appear most often in BI tools, CRM systems, or helpful hybrid reporting that spans these gaps.
Building trust in reporting starts with a few pillars or tenets:
Reports need to be timely, relevant, and accurate
Report definition and lineage (where does the data come from?) needs to be easy to understand and untangle
Data needs to be consistently explained over time
By delivering a metric or report that makes sense and is accepted by the team, you gain the ability to add more reports (creating a series of “one in a row” wins)
Let’s unpack each of these ideas to review the steps to get to reliable, consistent reporting. There are a lot of dimensions where this process can go sideways, and the way that you uncover and resolve anomalies goes a long way toward building trust in the reporting process, not just the reports themselves.
Reporting needs to be reliable and accurate
How would you define “reliable”? Reliable reporting means that you trust the outcome, even when it’s not an expected value. It means that you understand what the outcome means, can explain the cadence and definition of that outcome, and start to form an opinion on how to respond to an expected or unexpected result.
Accuracy defines whether the report is “right”. Simply put, if someone else calculated the answer to this question using the same methodology, would they not only get the same result (consistency) but can they also agree that the result is correctly calculated? For certain calculations, like the number of closed-won opportunities that happened in a date range, this is an easily verifiable number.
For other ideas, such as:
What is a customer?
How much attribution should we give to this marketing program?
Was this really a marketing-qualified lead?
Is this user a product-qualified lead?
Does this user have specific feature use that is higher than an average user?
We need a definition that can be objectively true or false, along with a methodology to count and prove that count.
What needs to be true for a great reporting outcome?
Data and GTM teams build trust in their data by agreeing on the definition and by clearly labeling the information so that when unusual information appears, it stands out. You can show that values are out of normal ranges by adding conditional formatting to reports (color coding them above and below certain thresholds).
In practice, this means:
Labeling the definitions for your terms
Ensuring that report consumers know how this data can be used effectively (e.g. it’s not possible to take hourly data from daily measurements, because the information is too small for the measurement)
Having a single place to review definitions (a Notion page, a field in the data that tells you what it is, or anything at all that points you in the right direction)
To solidify trust, data teams should make it clear what data ranges and time grains are available. You don’t want GTM teams looking at you confused when they ask for hourly averages but you have only daily data measurements to share with them. Trust is a two-way street. GTM teams also need a good understanding of what we’re measuring.
For example, to track customers from lead to opportunity to expansion, you will need to define the linkage between leads and accounts. This is usually done by matching converted leads to the accounts where they belong. If you’re managing expansion and it happens using a different contact at that same account, do you want to capture the account or the lead journey? They’re related, but not identical.
Consider “The Bowtie”, the data visualization technique shared by the Consulting firm Winning by Design. By aligning data for the business as interlocking processes that always need to share information, this diagram shows how reporting for each group needs to have a common foundation.
GTM teams need to learn the right way to make data requests. Giving data teams the right information to help you is one of the most important skills for GTM teams to learn.
As a GTM team member, if you start with questions like these when you want to ask questions about the data, it will help the data team deliver a meaningful report or metric:
What’s the outcome we’re trying to measure?
Do we have the data to answer this question?
What would it mean if this question were true?
What would we do based on this information, either to reinforce it or course correct?
Here are some examples of reporting to reinforce business goals.
A sales team wanted to improve their forecast accuracy, so they identified the steps of their funnel and the time between stages for in-funnel opportunities. This let them find the places in the funnel where opportunities were lagging, and also gave them a comparison point for segmented opportunities that won vs those that were closed lost.
A marketing team wanted to look at the initial conversion between a top-of-funnel form and prospects that eventually activated. By measuring the overall conversion and the time to activate, they were able to identify improvements to the nurture path that engaged prospects to take a first action.
A GTM team wanted to identify the success rate for prospects that used personal emails so that they could decide whether or not to require a business email for a registration flow. By measuring the prospect conversion by email domain type, they were able to give the business information to make this decision.
Everyone wants to use data to prove their work has a real impact on the shared outcomes of the business, but this isn’t always obvious. For GTM teams, this means the ability to connect the metric you are measuring to a business outcome that the business cares about. For Data Teams, this means making sure the data supports the ability to measure the items GTM teams have defined.
To convince teams that haven’t thought as much about the problem as you have, you need to show your work.
Documentation and showing your work
As a reporting consumer on the GTM or Data team, one of the most important things you can do is to familiarize yourself with the reports and what they’re telling you about the business.
There are a few conventions that help other people to orient themselves when they are looking for key information about reports and metrics.
Building a diagram to show lineage – or the way that input metrics inform other metrics later in a process – is one of the most important visuals you can build as a GTM team. By creating a visual representation of the “data as it works its way through the system”, GTM teams can get buy-in from their own team and other stakeholders while also explaining visually to the data team what they need to prove. Who owns this? It’s a shared responsibility between RevOps and Data teams, and usually starts with a process map.
For example, when you define a marketing-qualified lead, you need to create the actual steps and path in your head for this to be true. Whether the prospect enters the funnel by submitting a form, taking action, or a combination of several things, these are rules that need to be stated so that the data team can build them.
The first step to showing that work is to declare that there is an item that you want to track in the system, how it is defined, and how to validate it. These definitions are critical to gaining buy-in from other teams because they allow the teams to let you know if you have fundamental differences in the way you’re thinking about this information.
When you gain agreement on basic definitions for your data and your metrics, it’s possible to return to the data questions you asked previously to enable you to run the business and know if they are possible. For example, if you want to track attribution from website visitors through to authenticated users, you need to have some way to connect them. An IP address may not be enough to make the connection, but creating a way to resolve previously logged-in users to their web history might work.
The methods you use matter less than making sure there is a place where everyone who needs to know the definitions and details can review them. Think of this as a process of gaining trust by providing “one in a row” answers that reinforce the idea that you are working together.
Building a habit of quality and consistency
Create trust between Data Teams and GTM teams by creating a cadence for the delivery of high-quality output. This could start as simply as making a list of the reports that you are creating, the schedule on which they get created, and what they tell you.
By weaving the story of data creation into the regular life of the business, you will be building a habit of evaluating data and delivering results.
If you’re not already thinking about the policies and procedures for putting data quality and consistency into place, they might look like this:
Data governance policies - establishing who is able to change and update data, and what is the expected format for a “Minimum Viable Record” - or a record that can be used in the system instead of queued for remediation.
Data validation rules - what are the expected ways we will update data and validate that it looks the way we think it should look? This could dovetail with governance policies for items like picklists, standard opportunity stage names, and the like.
Scheduled audits - how often do we check to see if we’re creating new problems? You probably want to schedule this too
All of these habits start to build a culture of shared data success, where GTM and Data Teams are working together to create reports and data that are trusted, accurate, and interesting to the business at large.
What’s the takeaway? you can’t have reliable reporting without a known structure that helps you define, examine, and interpret your data in the context of the business. Building cadences to check that information adds to the growing trust between Data and GTM teams and helps them to build great things together.
Links for Reading and Sharing
These are links that caught my 👀
1/ A compendium of dataviz - Colin Megill shares a vast collection of resources on data visualization. The best piece here in my opinion is the curriculum for a course at the University of Washington on designing data visualization. You could stay busy and informed for a long time with this info.
2/ Creating analytics is more than point and click - Erghest Xheblati writes about the logical thinking process for analytics.
What I particularly like about this piece is the goal to step back and think about the larger problems we are trying to solve as analysts. You could have the correct answer to the exact wrong problem unless you frame what and where you are analyzing. This is a great resource for the next data exploration you do.
3/ Charts look better in vector - And when you need to place your data exploration in a presentation document, it will almost always look better when you’re visualizing it in something other than Google Sheets. Here’s a plug-in for Figma that builds charts from tabular data, including a nifty import straight from sheets. You’ll need to spend $20 to get full access to it, and it will be worth it after the first graph you create.
What to do next
Hit reply if you’ve got links to share, data stories, or want to say hello.
Want to book a discovery call to talk about how we can work together?
The next big thing always starts out being dismissed as a “toy.” - Chris Dixon