What is Data Operations, revisited
Data operations teams deliver data and insights for key business questions and enable operational changes based on the answers to those questions. Read: "Everything Starts Out Looking Like a Toy" #163
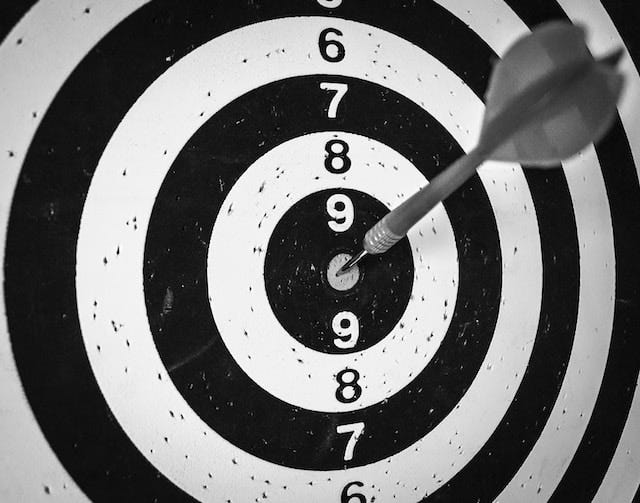
Hi, I’m Greg 👋! I write weekly product essays, including system “handshakes”, the expectations for workflow, and the jobs to be done for data. What is Data Operations? is a post that grew into Data & Ops, a team to help you with product, data, and operations.
This week’s toy: a browser-based economy simulator. Spend a little time thinking about the interplay of producers and consumers, then add some taxes and scarcity into the mix. It’s a fun rabbit hole into macroeconomics and data. Edition 163 of this newsletter is here - it’s September 18, 2023.
If you have comments or want to sponsor the newsletter, hit reply.
The Big Idea
A short long-form essay about data things
⚙️ Data Operations, revisited
When I started writing about data operations 162 issues ago I suggested an example definition that focused on data shared between systems, the people who update that information, the tools used to transform and transport data, and the governance process they used to keep things working.
That definition was functionally correct and was missing a critical element: what to do about the information you discover from that process.
Here’s an updated thesis:
Data operations teams deliver data and insights for key business questions and enable operational changes based on the answers to those questions.
What is Data Operations today?
Data Operations in 2020 was a nascent idea for how to build an internal data practice. I’ve been refining these ideas into everyday tasks and transforming the “inside a company version” of data operations into repeatable playbooks for improving operations.
What’s the Ideal Customer Profile for this data-improved enablement of operations?
FOR operations teams WHO want a cross-functional way to activate data AND want to drive change in their organizations, Data Operations is a style of working or a discreet team to find, understand, and troubleshoot data in the organization, usually to make Go-To-Market teams run faster and more efficiently.
Data Operations is not …
Data observability, though it uses those tools to provide insight
Data science, though it helps to have a sense of probability and statistics
Data engineering, but workflows, models, and continuous delivery of quality data is an important aspect of this practice
Data operations is a cross-functional idea to build products and services with data so that the whole company can run faster. It’s a force multiplier or flywheel to orient, observe, do, and adjust (OODA).
What does it look like in practice?
Data Operations is at the center of Ops Teams
The goal of an operations team is to help things run more smoothly inside of an organization. Whether that’s customer ops, sales ops, or some other kind of operations, they run regular plays that complete the essential shared support tasks in a company.
Support implies that things sometimes go awry. The ops team’s job? Fix it and get back on track while having minimal impact on the customer and internal team. That means finding the right resources to solve the problem, documenting it, and determining how to prevent it from happening again.
For these teams, Data Operations helps illuminate the problems between systems. When a workflow fails and sends an alert trigger to Slack; when a Dashboard doesn’t match expectations; and when stuff is jacked up, you need a team to solve that problem.
In the beginning, Ops teams self-service this troubleshooting. But as teams grow, it gets harder to think about the related network of possible things that could cause problems. Without a data ops mindset, making this repeatable is hard.
Data Operations is inherently cross-functional
This is a cross-functional role. That means you need to be an internal consultant to be effective in data operations, starting by following the data as it walks through systems and then identifying the failure points that happen.
Without knowing what’s supposed to happen and the normal workflow, it’s hard to build exception conditions and to alert when things break. When you take the time to learn how each team works, you’ll also see interesting threads across systems.
Some of these tools involve:
simply writing down what’s supposed to happen, including the expected data that a system receives
building process maps if you want to be fancy
documenting what happens today so that when things don’t work you have a record of making a mistake and don’t need to repeat it
If these things do not help other teams do their job better, they are fancy paperweight ideas. The purpose of a data operations team is to improve operations using the data that is already flowing through those systems.
Sometimes, the addition of a single field in an alert (or a link to another system) makes the process run better. In other cases, there might be a heavier lift. But the goal is to give the team superpowers.
Data Operations is a core business process
Data is the lifeblood of your business. And Data Operations can improve the business if you know what you are asking and why.
Take a typical metric: improving something from x to y by a specific date (e.g. improve the number of daily leads from 100 to 150 over a period of several weeks).
The metric is an outcome, but there are many inputs that cause that outcome to change. What goes into improving that number? Probably a series of efforts that improve both the top line of acquisition through a mid-funnel exercise to understand whether these leads convert better or worse than other leads through to the end conversion.
Moving a metric doesn’t guarantee success, so you need to know the outcome you need and the process that will help your team to observe, orient, do, and adjust.
Data Operations is the process of building playbooks that take you there.
What’s the takeaway? If you want to make iterative changes to your business and see whether they are working, Data Operations helps you take the sometimes complicated and confusing handoffs between teams and turn them into repeatable playbooks. By understanding what data needs to end up where, you can make things better for the whole team.
Links for Reading and Sharing
These are links that caught my 👀
1/ First principles for jumping bugs - It’s all the rage to think batteries are the best way to power devices (and vehicles) these days. But what if controlled combustion could be used in a different form for robotics? If you could handle the emissions, it might be a bridge technology from current battery power/weight ratios to the future.
Here’s a jumping “bug” powered by combustion …
2/ How an engineer built an Excel clone at Uber - This is a wonderful story about the creativity of engineers, and how a great idea might only see the light of day for a little while. It also gives you a glimpse into the engineering culture at Uber.
3/ Use AI as a regular tool - If you’ve been wondering about the relative performance gains for AI in regular knowledge work, this study supports the conclusion that yes, AI is here to stay. Workers who use some form of AI on tasks perform better. Does this mean you should let the AI do the work for you? Not really - it means it’s going to be your exoskeleton as you move faster and farther.
What to do next
Hit reply if you’ve got links to share, data stories, or want to say hello.
Want to book a discovery call to talk about how we can work together?
The next big thing always starts out being dismissed as a “toy.” - Chris Dixon