Adding time travel to your GTM analysis
How do you level up your GTM from knowing what's happening right now? Knowing what happened at a point in time. Snapshots make that possible. Read: "Everything Starts Out Looking Like a Toy" #189
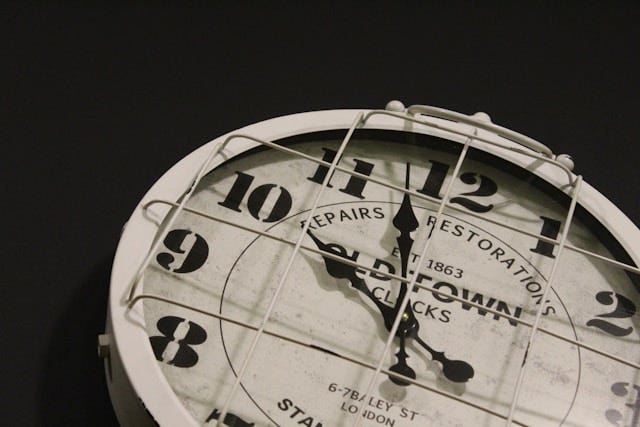
Hi, I’m Greg 👋! I write weekly product essays, including system “handshakes”, the expectations for workflow, and the jobs to be done for data. What is Data Operations? was the first post in the series.
This week’s toy: playing volleyball with Pikachu in the browser. Why don’t they make more simple games like this one? Casual games are fun, and you don’t have to spend hours learning how to play. Edition 189 of this newsletter is here - it’s March 11, 2024.
Brought to you by Apollo.io, an all-in-one solution providing RevOps teams with access to data, enrichment, outreach, call intelligence, scoring, calendaring, and email automation. They integrate seamlessly with your CRM and existing workflows. The quality of Apollo’s data is unmatched, they are ranked #1 for contact and company data accuracy on G2 with over 6000 reviews and a 4.8 star rating. Learn more …
If you have a comment or are interested in sponsoring, hit reply.
The Big Idea
A short long-form essay about data things
⚙️ Adding time travel to your GTM analysis
A key problem in every GTM organization is understanding: “what’s happening right now?” Once the team gets that answer, they want to know what will happen next. The best information you have to answer that question is to look at the history of what’s already happened.
You need to forecast the future by looking at the past. It’s not an exact science, but looking at what happened at a point in time, especially a point in time in a cohort, can give you clues to the future.
Example: Rollup fields
Rollup fields help you get the current state of related data, answering questions like “how many contacts does this account have right now?” By taking related queries and running them in the background, a rollup field makes it easier to conduct complicated analysis.
You can also create segments in your data by asking true/false questions about these rollup fields, e.g.
does this account fall into the ideal customer profile?
has the account passed an activity threshold this week?
when enriched, is the account in a target industry?
All of these questions are a good way to bucket accounts into dynamic groups right now. But one of the things you’ll want to know about calculations like this one is how they change over time.
Why track change over time?
When you’re thinking about slowly changing dimensions, especially ones that involve counting other things like pipeline, cumulative activity, or segment membership, storing this information in snapshotted tables will help you to see this change on a daily, weekly, or monthly basis.
Using a snapshot lets you zoom backward to a point in the past where you can apply existing or new logic based on the fields you captured at the time. Whether you’re referring to something concrete – the count of accounts in a particular stage and the qualified opportunity dollars in pipeline on a particular date – or something more abstract, like segment membership, snapshots show future you what was happening at that date and time.
Time grains and storing snapshot data
After the data is stored, you don’t typically get to go back and recapture it, so be careful to select the initial time grain to store the data. Daily data is a good middle ground to capture that gives you later flexibility while minimizing the number of rows stored. It also makes it relatively easy to transform measurements into weekly, monthly, quarterly, and annual increments capturing incremental change.
For our segment example, you might model the data this way to capture the movement of accounts from segment to segment over time:
a segment table, with ID, segment name, description, start date, and end date to capture the slow changes of your segments
a snapshot table with segmentID, accountID, start date, and end date, event, event description (along with any other relevant account fields)
an account snapshot table captured daily with account fields
This structure could give you changes over time relative to the account and its movement in and out of segments, revealing both the change in the account and the change in the way you are thinking about it over time. By using both of these change sets, you make it possible to track arbitrary changes in your data, while also enabling backfilling of segment data if you recombine changes in your snapshotted accounts later.
Why note when an account enters or leaves a segment?
Segments – groups of records that meet a criteria – are shorthand for steps in the customer journey. Whether you are using this idea to track “frequent buyers”, “trialers that did not convert”, “accounts that are customers for more than 18 months”, or different kinds of measurements, this is a great way to market different messages to different accounts.
By understanding when accounts enter or leave segments, you have a better idea of the composition of that cohort of accounts, and can answer questions like:
for the group of trialers that converted this month to paying accounts, what were they doing on days 3-8 in their trial?
how does this cohort (segmented) compare to past accounts in that same group?
did they become active immediately, or did they take an action later
Segment information is important to understand how customers develop, especially when you’re going to show a slice of that over time.
Snapshots and BI Tools
The change of rolled up fields along with the change of non-rolled up fields gives you history to display in your Business Intelligence tools. If you have to pick one set of snapshots to capture, use the raw data because you can aggregate and roll it up later. Ultimately you’re going to want to answer the question of “how many accounts were early stage and how many were late stage on a date.”
You’ll also want to know which ones became closed won accounts, which abandoned, and perhaps which ones came back later as part of another sales cycle. Building snapshots of slowly changing data, especially the changes in roll-up fields, makes that possible.
What’s the takeaway? The current state of your GTM tools doesn’t tell you much about the history of the data in the system. When you start capturing snapshots, you enable time travel for your data.
Links for Reading and Sharing
These are links that caught my 👀
1/ Cultivate a bias for action - This quick essay by Cate Hall offers practical advice on how to be more agentic. It’s a fancy way of saying, “when no one tells you what’s important, figure out what’s important and go do that.” I enjoyed this as a set of steps you could throw at any problem to get unstuck.
2/ Then what? - It would be nice if having more data told you what to do. Mikkel Dengsøe recommends following your intuition, making small bets, and measuring the results. It’s not surprising that it’s the method most people would tell you to follow to validate your data. However, saying it (or writing it) out loud reminds us that data is as much art as science some days.
3/ Spring forward and your brain - If you’re still feeling foggy from the effects of the time change at 2am yesterday, you might want to take it easy for a few days. Changing the time knocks your brain for a loop.
What to do next
Hit reply if you’ve got links to share, data stories, or want to say hello.
The next big thing always starts out being dismissed as a “toy.” - Chris Dixon